The Analyst’s Corner: How to Benchmark Engaged Time
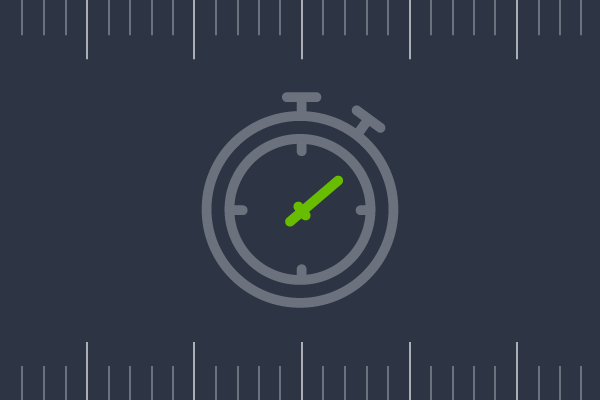
“What if we stopped focusing so much on traffic, and started focusing on experience?” I’m asked this question frequently. It’s a complex, divisive challenge for newsrooms, one I eagerly indulge.
I work directly with media companies of all shapes and sizes. I offer consultation on everything from using data to support editorial intuition, experimenting with audience development projects and honing tagging strategies. The best newsroom strategies start with someone saying to me: “It would be so interesting if we could…” and “What would happen if we tried…”. Data can provide the foundation for sussing out those new ideas while mitigating risk.
When I start to talk to clients about using engaged time, or any metric for that matter, the key is to set goals. What does success look like? But this prompts new questions:
How do we stack up? Is this kind of engagement normal? Is it good? For us? For anyone?
There is no reliable comScore or Alexa rating for attention, thanks in no small part to various ways of measuring “time on site.”
So if a publisher is eager to change the way they think about their audience, and their success, where could they start? I tried to find out.
How does Parse.ly measure engaged time?
First, let’s clarify exactly what we’re talking about, because Parse.ly measures engaged time differently than other analytics platforms. On your site, a visitor is considered “engaged” if they 1.) Have a browser tab open, and 2.) take an action (scroll, click, mouse-over) at least once every ten seconds.
For more details, feel free to browse this explainer on Parse.ly engaged time, or for a more technical explanation, read our engaged time technical documentation.
Analysis 1: How do we find an average engaged time for all content?
I first set up an exploratory analysis across our network, to see if I could identify any clear patterns. Over time, I started to see the same sites consistently outperform other outlets in average engaged time per visitor. What initially struck me though was actually the lack of pattern: each site seemed so diverse in voice and size.
To identify what made them competitive, I needed to understand the bigger picture of engaged time across Parse.ly’s network. To do this, I sampled user experiences at random for content published within one month from 300 anonymized domains to first understand, “how many seconds can you expect a typical reader to remain engaged with a story?”
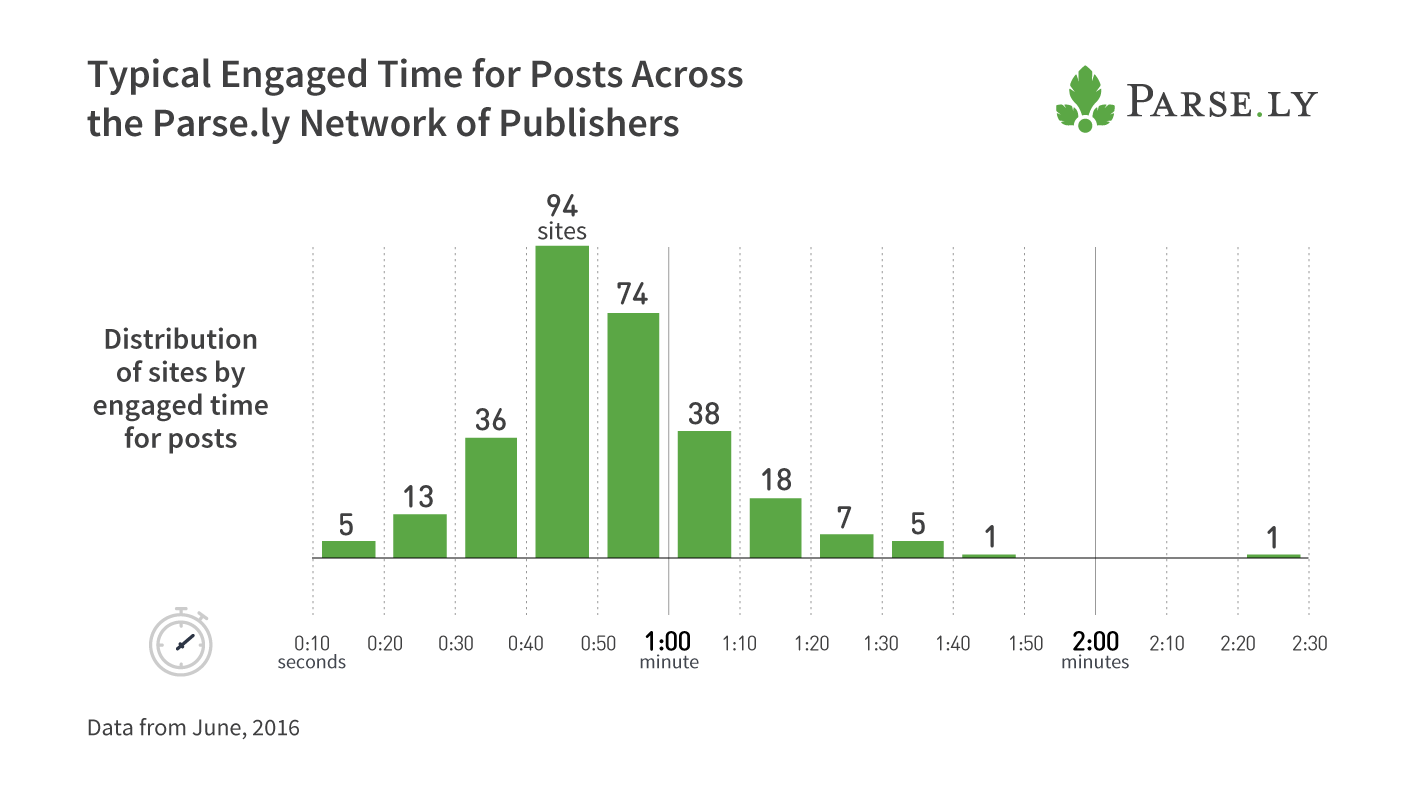
Here we see how attentiveness is distributed across Parse.ly’s network. This graph shows what we can expect for “normal” attention time from any given reader, to any given article for each of the sampled domains. You can see a majority of our publishers attract an audience willing to invest roughly 40-60 seconds of their time on an article, though plenty of publishers can expect a more invested audience.
This provided a starting point for benchmarking engaged time, especially for my clients. Any Parse.ly user can easily find in their dashboard where their site, section or article falls on this curve. Teams can check to see if their stories and authors fall above or below the norm for their audience.
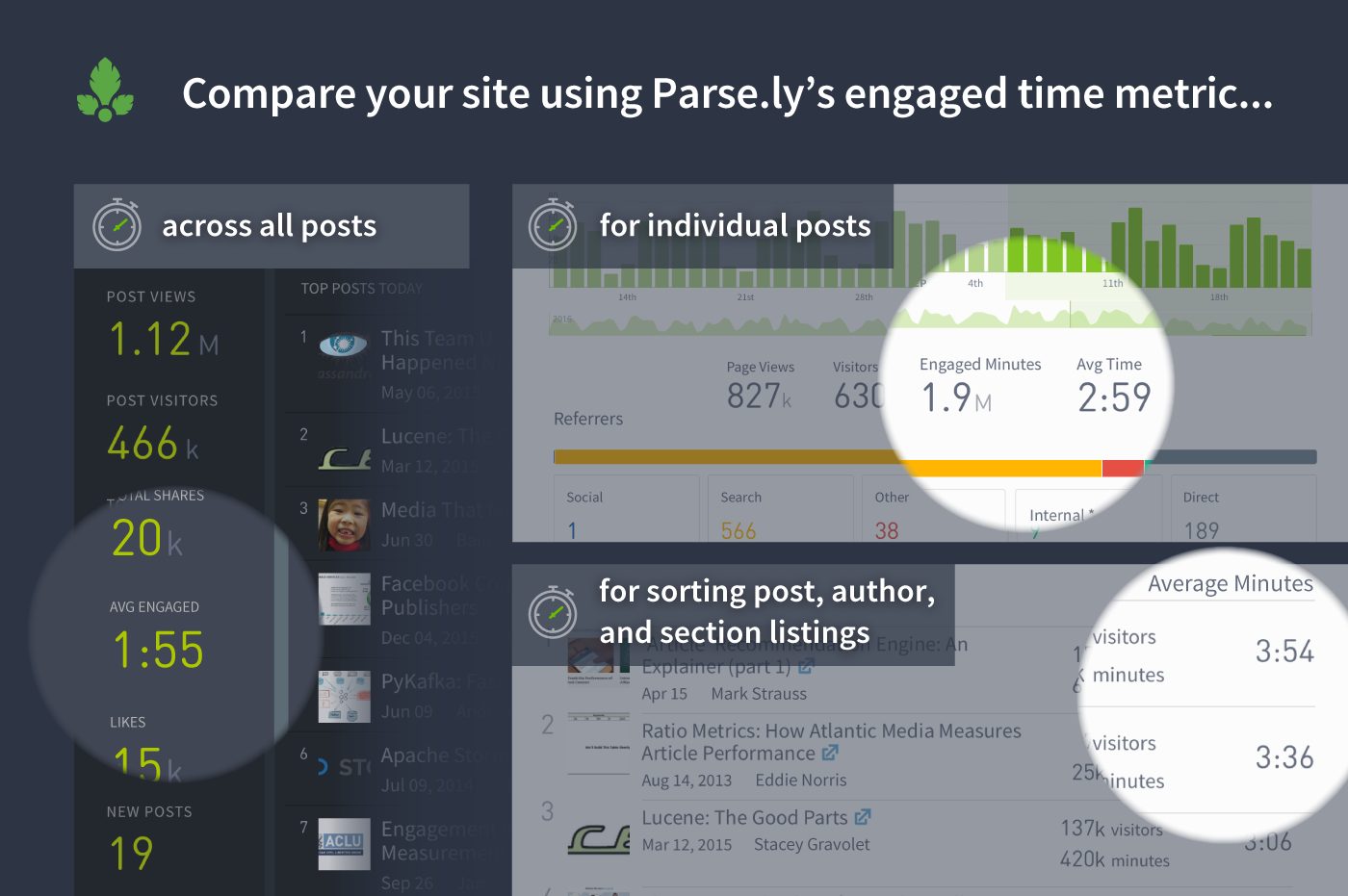
Understanding the context here is crucial though; not every article needs to outperform the average of the Entire Internet. There are better questions to ask. Where does your article fit in relation to what is expected for its section? How does that section perform in relation to the site as a whole?
Of course, there’s one more comparison that everyone wants to make: how does my site stack to the competition?
Analysis 2: How do we find the averaged engaged time for similar content?
This question helps you contextualize whether your work gets more attention than pieces that are similar to it and potentially predict how other topics, outside of your core competencies, might perform. For example, if you don’t normally write technology features, it could help to understand the engaged time benchmark for tech publishing leaders. Here, we break down the analysis above further to understand how long a reader could be engaged on similar content.
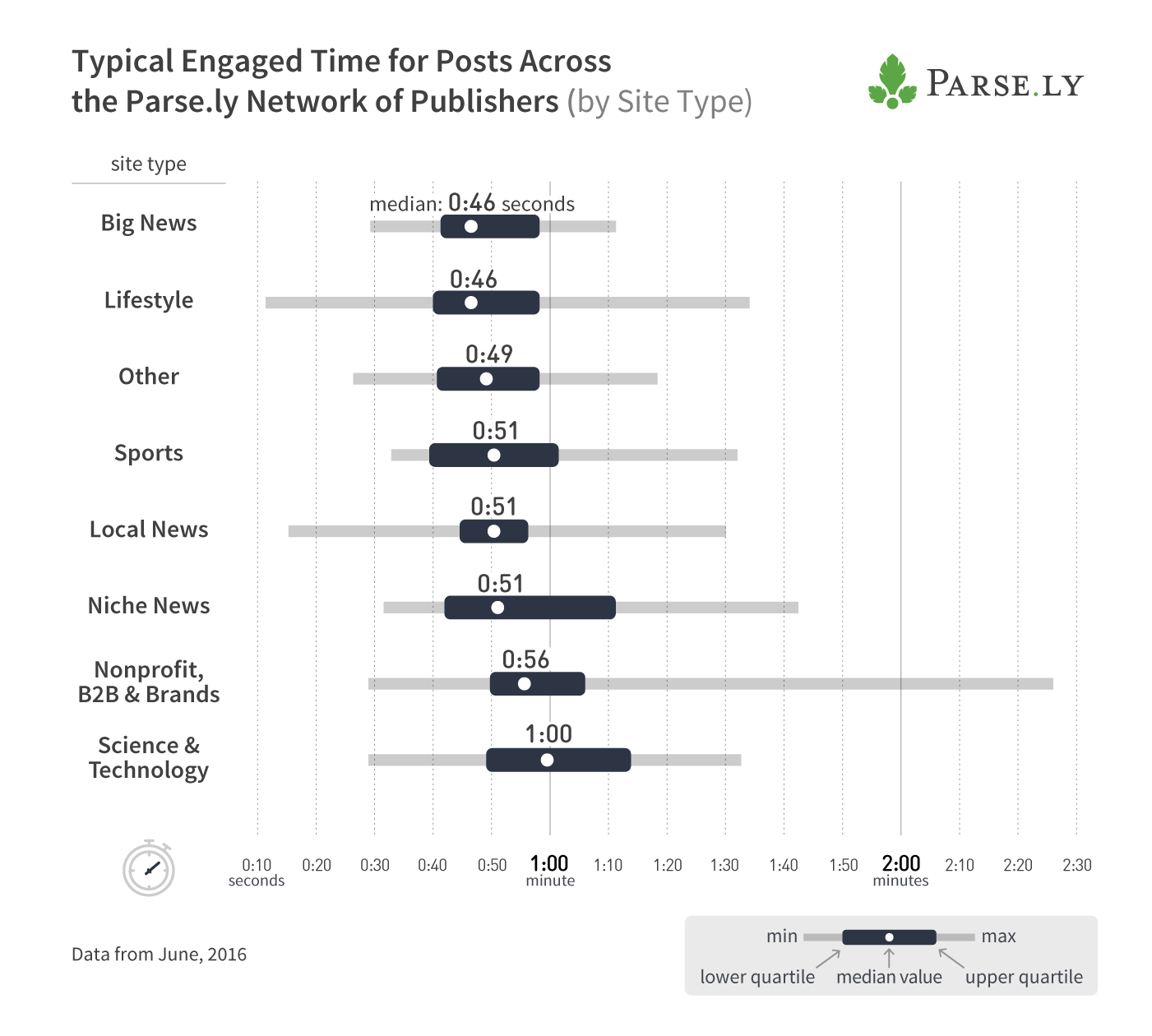
Broken out by publisher type, it’s easy to see how nuanced attentiveness is across different types of content. I found it noteworthy that local news sites command more engagement than major news outlets, even with undoubtedly fewer resources.
I mentioned earlier when I began investigating engagement, I was struck by how, month after month, the same set of publishers kept leading the pack, albeit with no discernible pattern among them. Broadening the analysis to the network, broken down by these categories, most of these names resurfaced at the top of their respective categories. A pattern finally became clear: the most engaging sites within each type of publisher were highly recognizable brand names.
Also, now that we’ve broken out engaged time averages across Parse.ly’s network, it’s easy to see how using homogenized data from a heterogenous group of sites to set benchmarks could do more harm than good. Certainly, the same concept applies within the newsroom; measuring the average engaged time on an article against what is expected for that vertical or topic will provide better context.
What do we know about engaged readers?
We’ll continue to explore other patterns within the most engaging experiences. In the meantime, here’s a reminder of what we do know:
- Facebook vs Twitter. We already know from a recent study with Pew Research that, in an increasingly mobile ecosystem, referral sources on mobile were an important factor in determining engaged time. Their research found that Tumblr and Twitter generate highly engaged audiences, while Facebook audiences were less engaged.
- Readers can be highly engaged on mobile, though infrequently. In another section of the same report, we distinguished between long-form articles with 1000+ words and short-form articles. Long-form consistently outperformed short-form, though visitors to either do not frequently go on to other articles.
- In the analysis conducted for this post, we found no correlation between page views and engaged time. A large audience is not necessarily an attentive one.
How to navigate this brave new world
We’ve found ourselves in somewhat uncharted territory in an effort to shift away from primary traffic metrics like page views and unique visitors. How do we define what makes something “good” anymore? Why should we care about engaged time at all?
As I found in this analysis, the engaged time metric provides us an interesting exploration in how we can set a more relevant benchmark. In clinging to familiar traffic indicators like page views, perhaps we have systematically neglected not only the experience of our readers, but the nuance of our reporting. But coupling traffic metrics with engaged time helps us understand which articles create the most impact.
If your post gets hundreds of thousands of viral views, but no one sticks around to read it, did you really manage to convey anything meaningful? Increasing your newsroom’s dedication to understanding the relevancy of engagement in all its forms will lead to a deeper, better audience strategy.